Part 1: Defining the Problem to Optimally Balance Competing Requirements
Note: This post is the first of a three-part series that will examine the question “What is the most effective fleet management strategy based on data-driven decision making?” In part one, a mental model is defined to help program managers understand the trade-offs they may face. (Read part two, part three).
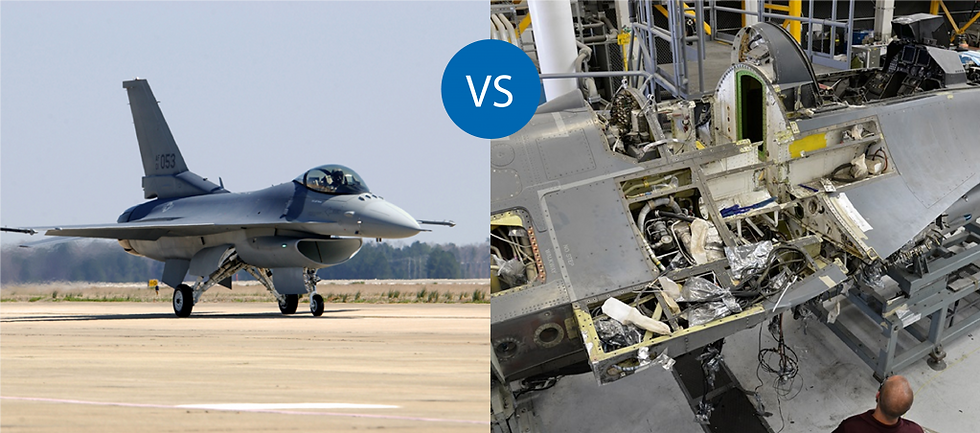
(U.S. Air Force photos/Staff Sgt. Keri S. Whitehead & Alex R. Lloyd)
Effective fleet management is an exercise in managing competing priorities. Operators demand availability to meet mission and training requirements and capability necessary to maintain technological relevancy, ensure survivability, and achieve mission effectiveness. Juxtaposed with availability and capability demands is the demand for safety—achieved, in part, through required heavy maintenance. Changing threats, new missions, and fleet age make the competition among these priorities all the more dramatic.
Enter the program manager. In close consultation with the program’s stakeholders—operators, maintainers, requirements personnel, contractors, reliability and maintainability engineers, and depot planners—the program manager must find the optimal balance within a very complex problem. The question becomes:
What is the most effective fleet management strategy based on data-driven decision-making?
Working within a Complex, Multi-variate Trade Space
Making sound trade-offs between competing fleet management priorities requires two things. First, the fleet management problem must be broken down to a level of indenture permitting understanding of variables and their interactions. Second, these variables must be selected and defined in ways that can be measured, thereby facilitating analysis and data-driven decision-making.
Certainly, there are several ways of thinking about fleet management which satisfy both requirements. One such “mental model,” presented in the following diagram, depicts the trade-offs between competing fleet management variables. It is presented in terms of eight “measures of merit,” each defined in quantitative terms. Each measure is also identified in terms of what is being optimized—that is, minimized or maximized. Taken together, these measures form a multi-variate, multi-dimensional trade space.

Starting at the top of the diagram and proceeding clockwise, the definition of each measure of merit follows:
Minimum essential depot production footprint: the minimum essential number of hangar bays/ramps spots, support equipment, and maintenance personnel required to meet depot production requirements (heavy maintenance or modification work or both); minimizing this measure lowers the risk of capacity constraints.
Minimum aircraft induction engineering waivers: the minimum number of engineering waivers required to bring an aircraft into depot maintenance early or extend the aircraft beyond its scheduled induction in order to minimize the number of aircraft in queue at the depot; minimizing this measure maximizes aircraft availability and reduces the risk of catastrophic mishaps and fleet grounding.
Maximum aircraft availability: the maximum number of aircraft available; achieved by minimizing aircraft in work at the depot or by contractor field teams(CFTs); maximizing this measure increases the likelihood of fully meeting mission and training requirements.
Minimum essential CFT production footprint: the minimum number of essential hangar bays/ramp spots, support equipment, and maintenance personnel required to meet CFT production requirements (heavy maintenance or modification work or both); minimizing this measure lowers the risk of capacity constraints and interference with operations.
Lowest cost: the minimum amount of financial resources required to execute all depot and CFT work.
Earliest modification IOC/FOC attainment: the minimum time required to reach initial/full operational capability (IOC/FOC) for a particular modification; minimizing this measure increases the ability to meet mission requirements.
Maximum CFT production stability: the maximum degree of regularity in meeting aircraft scheduled induction dates for CFT work; maximizing this measure reduces CFT production schedule perturbations.
Maximum depot production stability: the maximum degree of regularity in meeting aircraft scheduled induction dates for depot work; maximizing this measure reduces depot production schedule perturbations.The trade space diagram yields several observations.
Along any particular measure of merit vector, we move out to that measure’s optimal condition as we move away from the center.
However, doing so will leave all other measures at their greatest sub-optimization (a value of “zero”).
The challenge then is to find the point within the trade space where an aggregate maximization of all variables is reached–each measure is either maximized or minimized in relation to the constraints placed on it by the other measures.
This, or any mental model, is instructive and necessary. It’s instructive in that it segments a very complex problem into sections or aspects that can be understood, and identifies where interrelationships between sections can be conceptualized and from which data can be collected. The mental model is necessary in that it forms the foundation for practical model development and the application of analytics to turn conceptualization to data-driven insights.
Dayton Aerospace Support
Dayton Aerospace is well positioned to help aircraft programs address their fleet management challenges. Our analytics and product support subject matter experts (SMEs) have successfully performed multiple analyses assessing the trade-offs between competing priorities by integrating depot maintenance and aircraft modifications to optimize aircraft availability. We have substantial experience tackling the phases of the analytics process—problem definition; business and analytic objective definition; ground rules and assumption identification; data definition; data collection and integration; analysis and results interpretation; results evaluation against objectives; and communication and deployment of recommendations. In doing so, Dayton Aerospace provides customers with data-driven decisions and the analytics necessary to perform additional inquiries. Our approach to effective fleet management utilizes the high value, authoritative sources of system data and models described in the Air Force Research Laboratory’s (AFRL) Digital Hangar as a continuum across disciplines to support effective fleet management strategies.
Part two of this series will present a modeling construct to analyze alternative scenarios that explore trade-offs between competing priorities. The third and final post will expand the question to the depot enterprise-level, presenting an approach to effectively address future capacity in consort with fleet management plans.
About the Author
Brian Waechter, PfMB, LSS BB, Colonel, USAF (Ret) has over 35 years of experience as a senior acquisition, logistics, and maintenance officer; defense industry vice president and business unit leader; operations research analyst; and Lean Six Sigma Black Belt. He has helped numerous programs analyze complex fleet management challenges and develop effective fleet management strategies that successfully balance multiple program trade-offs. Read more>